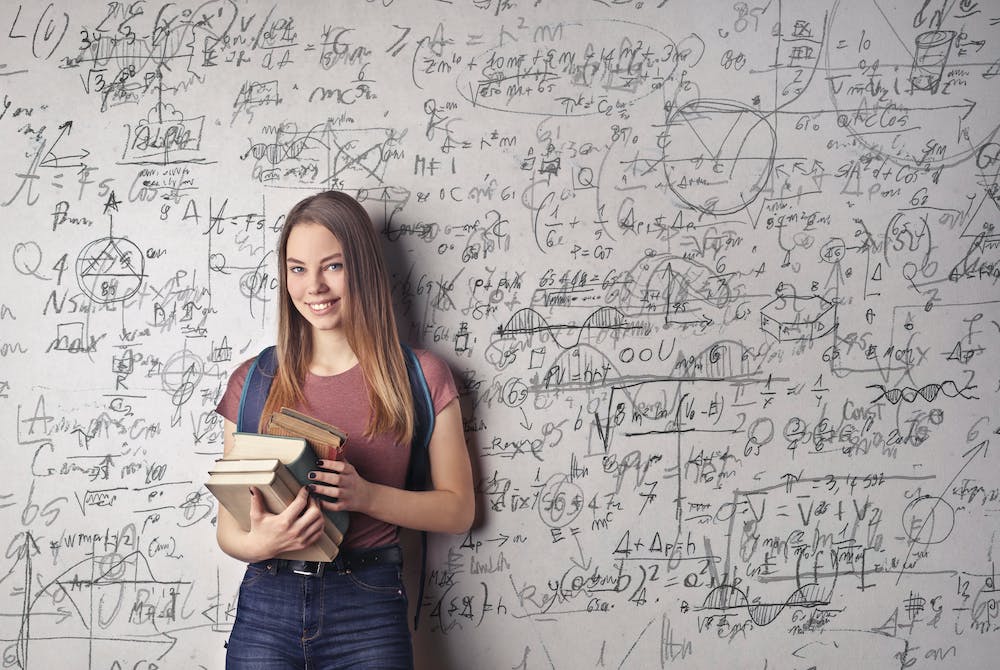
The rapid evolution of generative AI has unlocked vast opportunities for businesses. However, as marketers and product managers envision new features leveraging this technology, they must carefully assess the intricacies of implementation. Two predominant factors come into play: complexity and security. To prioritize new applications or features, a scoring system that rates both the complexity of development as well as the data security required can be useful. Here’s one way to develop a complexity-security matrix for scoring these types of applications.
Complexity in Generative AI Implementation
Complexity stems from the depth of customization and the sophistication of the AI model you aim to deploy:
- Public, Consumer Apps: These are off-the-shelf tools, often with limited customization, serving broad purposes. Examples include automated content generators or basic chatbots available on app stores.
- Enterprise SaaS Offerings: Designed for business purposes, these come with better customization and integration features. They might include CRM tools with AI-powered sales prediction features.
- Pre-trained Models: Models like Anthropic’s Claude 2 that come pre-trained and are ready to deploy. They offer generalized solutions and can be integrated into specific applications with minimal adjustments.
- Fine-tuned Models: These are pre-trained models further refined on a specific dataset to cater to niche tasks. An example might be fine-tuning for legal or other industry jargon.
- Self-trained Models Built from Scratch: The most complex, these are bespoke models, built and trained for highly specific applications. They demand significant expertise, data, and computational resources.
Many offerings in the first couple of categories can be deployed quickly, even by non-technical users. The more sophisticated offerings require more development, more time spent training the application to deliver the expected results, and in-house teams or third-party vendors with specialized skills.
Security Considerations
Security considerations will revolve around the nature of data the AI model will interact with:
- Publicly Available Data: Information freely available on the internet, like Wikipedia articles or open forums, or information your company already publicly shares, like help documentation or product manuals.
- General Business Data: Non-sensitive company data that might be proprietary but isn’t regulated or particularly vulnerable.
- Customer Data: Personal data from customers, such as their previous purchases, which, if mishandled, can lead to customer trust issues but isn’t specifically legally protected.
- Sensitive Customer Data: This includes financial details, addresses, and the like – the mishandling of which might have broad legal implications.
- Highly Sensitive and Regulated Data: Health records, biometrics, or customer data that falls under stringent and specific privacy regulations like GDPR or HIPAA.
The Complexity-Security Matrix
To aid decision-making, we propose a matrix. Envision it as a grid. The x-axis represents complexity, moving from consumer apps to self-trained models. The y-axis represents security, ranging from public data to highly sensitive data. Here’s how to score:
- Score Complexity:
- Public, Consumer Apps = 1
- Enterprise SaaS Offerings = 2
- Pre-trained Models = 3
- Fine-tuned Models = 4
- Self-trained Models = 5
- Score Security:
- Publicly Available Data = 1
- General Business Data = 2
- Customer Data = 3
- Sensitive Customer Data = 4
- Highly Sensitive and Regulated Data = 5
Plot your envisioned AI feature on this graph. A feature falling in the bottom-left is relatively easy to implement with minimal security concerns. As you move towards the top-right, the challenges increase in terms of development time and skill as well as privacy and legal concerns. This assessment makes it easier to prioritize quick wins, understand when to bring in additional development and legal resources, decide which features should require customer opt-in, and make business decisions for prioritization. For example, if a feature has a high expected return on investment and scores in the bottom left, it’s likely to be a quick win over a feature with similar expected ROI but in the middle of the chart.
Conclusion
As AI continues its foray into the business domain, its potential has captivated businesses to envision powerful, personalized features that leverage its power. However, a careful assessment using tools like the Complexity-Security Matrix ensures that the excitement of innovation doesn’t overshadow the essential considerations of feasibility and security. By straddling the balance, marketers and product managers can optimally harness the power of generative AI.